アプリ関連ニュース
- 2024年3月26日
- AI
Copilot key on Microsoft’s new Surface devices
Microsoft is gearing up for its Build event with a preview online event, shifting the spotlight to AI advancements, particularly through its Copilot feature. The unveiling of the Surface Pro 10 for Business and Surface Laptop 6 for Business at this event puts Copilot front and center, with a dedicated key embedded in the keyboard. This move underscores Microsoft’s commitment to integrating AI into its hardware, making tasks like planning, document retrieval, and website analysis more accessible to users with a simple button press.
These new Surface PCs mark a significant step in Microsoft’s journey towards what they’re terming as “the first Surface PCs optimized for AI.” By incorporating Copilot directly into the keyboard, Microsoft demonstrates a deep investment in leveraging AI technology. While it’s still relatively early days for Copilot, with its launch just a year ago, this move signals a strategic alignment with the burgeoning trend of AI integration in computing devices. Despite the past presence of Cortana keys, Microsoft’s focus on Copilot underscores its dedication to enhancing user experiences through AI.
The industry at large has seen a surge in branding around AI, with terms like “AI PC” and “AI smartphones” becoming commonplace. While these labels may seem abstract to consumers, they reflect a broader push towards AI-powered functionalities. Microsoft’s approach of branding these Surface devices as “optimized for AI” strikes a balance between acknowledging the trend and providing tangible benefits to users. Ultimately, while a Copilot key may seem like a small addition, its presence underscores Microsoft’s commitment to integrating AI seamlessly into everyday computing tasks, all while respecting the constraints of physical device design.
Yuuma
yuuma at 2024年03月26日 10:00:00
- 2024年3月12日
- 技術情報
Differences between C and Go programming languages
In the realm of programming languages, each one comes with its own set of strengths and characteristics, tailored to suit different needs and preferences of developers. Two prominent languages that often find themselves in comparison are C and Go (or Golang). Let’s delve into the key differences between these two languages across various aspects.
1. Memory Management
– In C, developers need to manually manage memory, allocating and deallocating it for variables. This hands-on approach gives precise control but also opens doors to memory-related bugs.
– Go takes a different route with automatic garbage collection, simplifying memory management tasks for developers and reducing the likelihood of memory leaks.
2. Concurrency
– Go shines in this department with its built-in support for concurrency. Goroutines and channels make it easier to write concurrent programs without worrying about low-level threading intricacies.
– C, lacking built-in concurrency primitives, often resorts to external threading libraries like POSIX threads, which can be more complex and error-prone.
3. Type System
– C boasts a weak static type system, allowing implicit type conversions and offering limited type checking.
– Go’s strong static type system enhances type safety and reduces common programming errors by enforcing stricter type rules.
4. Error Handling
– Go introduces a unique error handling mechanism using return values, eschewing exceptions. This approach encourages explicit error handling, leading to more robust and predictable code.
– C relies on error codes, return values, and functions like perror() for error handling, which can be less structured and more error-prone compared to Go’s approach.
5. Standard Library
– C’s standard library is minimalistic, often necessitating reliance on third-party libraries for additional functionalities.
– Go boasts a rich standard library covering a wide range of tasks, from networking to encryption, reducing the need for external dependencies and streamlining development.
6. Memory Safety
– C’s flexibility comes at a cost, as direct memory manipulation can lead to common pitfalls like buffer overflows and dangling pointers.
– Go prioritizes memory safety, employing automatic bounds checking and garbage collection to mitigate memory-related bugs, enhancing overall code reliability.
In conclusion, while C and Go share some similarities, they diverge significantly in their approach to memory management, concurrency, type systems, error handling, standard libraries, and memory safety. Understanding these differences can help developers make informed decisions when selecting the right language for their projects, based on factors such as performance requirements, ease of development, and code reliability.
Hope you enjoy that.
Asahi
waithaw at 2024年03月12日 10:00:00
Apple is making easier for users who want to switch to Android
Apple’s announcement regarding its plans to facilitate the transition of users from iPhones to Android devices marks a significant response to the EU’s Digital Markets Act (DMA). With the DMA in effect, Apple is compelled to enhance data portability and competition within its ecosystem. By committing to developing a user-friendly process for transferring data from iPhones to non-Apple devices by fall 2025, Apple aims to meet the requirements set forth by the EU while also navigating potential financial impacts on its business. Despite the introduction of new fees for developers who opt for DMA rules, Apple’s strategic maneuvers demonstrate its adaptability to regulatory changes while maintaining its market position.
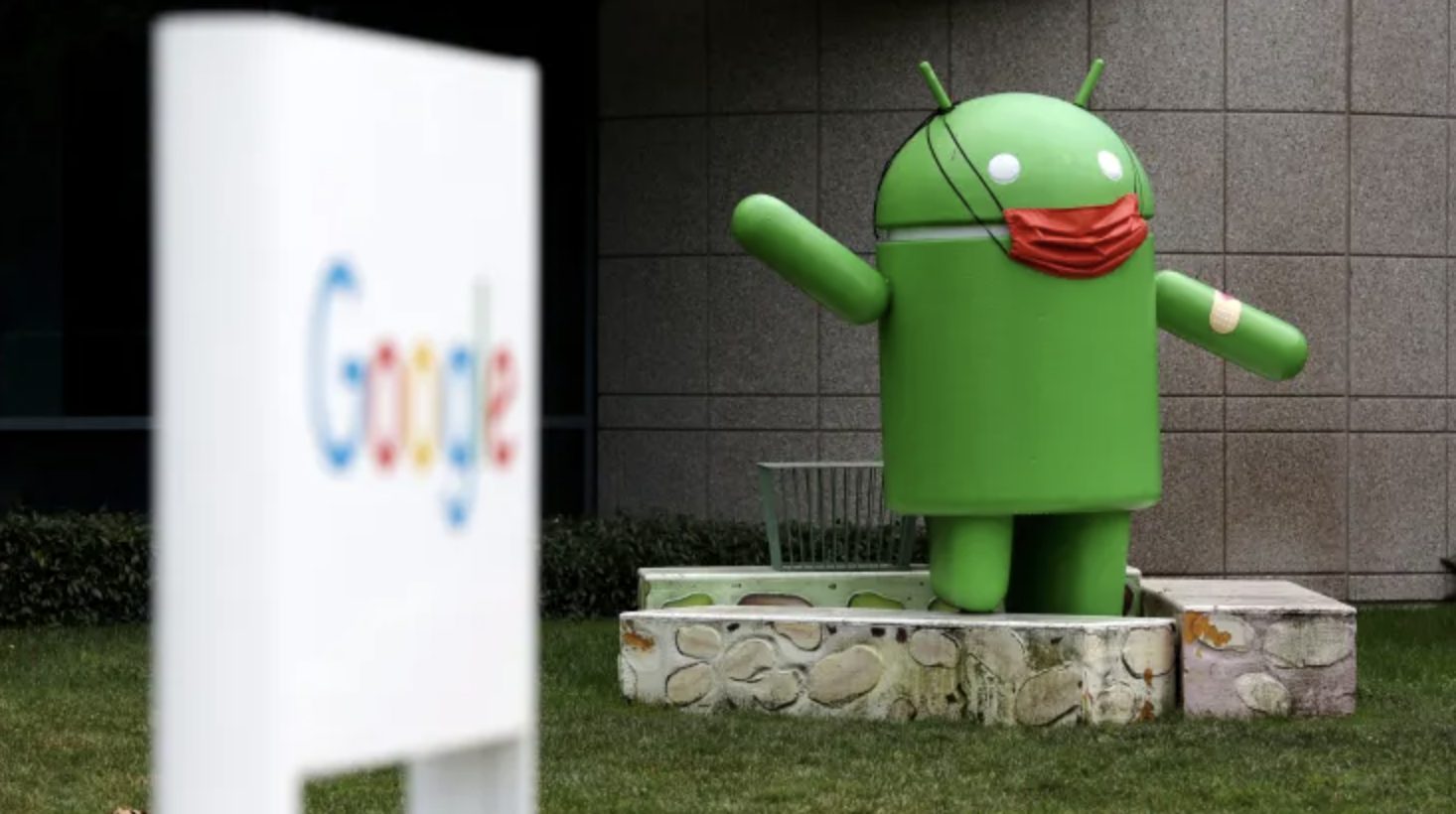
The expansion of data portability options, including the ability to export App Store data to authorized third parties, reflects Apple’s efforts to comply with the DMA’s provisions. Through updates to its Data & Privacy website, users gain greater control over their data, with options to transfer various types of information, such as photos, contacts, and browser data. Additionally, the scheduled downloads feature enhances user convenience by providing access to updated data over specific timeframes, ensuring the continuity of information across platforms.
As Apple continues to refine its data portability offerings, the focus on facilitating transitions to non-Apple devices underscores the evolving dynamics of the mobile ecosystem. While specifics about the new solution remain undisclosed, Apple’s commitment to assisting other mobile operating system providers in building seamless data transfer mechanisms suggests a broader industry shift towards interoperability. Ultimately, as regulatory pressures drive innovation and competition in the app ecosystem, users stand to benefit from increased choice and control over their digital experiences, regardless of the platform they choose.
yuuma at 2024年03月11日 10:00:00
- 2024年2月29日
- AI
Google AI Studio 使用方法
nishida at 2024年02月29日 10:00:00
Streamlining Electron.js App with Tray Icons
In the realm of desktop application development, Electron.js stands out as a robust framework for building cross-platform apps using web technologies. Among its many features, integrating tray icons can significantly enhance user experience by providing quick access to essential functionalities. Today, let’s explore how we can implement tray icons in our Electron.js application, from setup to implementation.
Setting Up A Electron.js Project
First things first, let’s set up a basic Electron.js project:
1. Initialize a new Node.js project
mkdir project_name && cd project_name
npm init -y
2. Install Electron.js
npm install electron
3. Create project files
project_name/
├── icon.png
├── index.html
├── index.js
├── node_modules
├── package.json
└── package-lock.json
– `index.html` for the application’s UI.
– `index.js` for Electron main process code.
– An icon file (`icon.png`) for the tray icon.
– add `start` command in package.json like this.
{
"name": "project_name",
"version": "1.0.0",
"description": "",
"main": "index.js",
"scripts": {
"test": "echo \"Error: no test specified\" && exit 1",
"start": "electron ."
},
"keywords": [],
"author": "",
"license": "ISC",
"dependencies": {
"electron": "^29.0.1"
}
}
Implementing Tray Icon Functionality
Next, let’s dive into implementing the tray icon functionality in `index.js` file:
const { app, Tray, BrowserWindow } = require('electron');
const path = require('path');
let tray = null;
let mainWindow = null;
app.on('ready', () => {
tray = new Tray(path.join(__dirname, 'icon.png'));
mainWindow = new BrowserWindow({
width: 800,
height: 600,
show: false,
webPreferences: {
nodeIntegration: true
}
});
mainWindow.loadFile('index.html');
tray.on('click', () => {
mainWindow.isVisible() ? mainWindow.hide() : mainWindow.show();
});
});
Running Your Electron.js Application
To run Electron.js application, execute the following command in the terminal:
npm start
This command will start Electron application, and we should see the tray icon appear in our system tray. Clicking on the tray icon should toggle the visibility of the application window.
Conclusion
Integrating tray icons into Electron.js application is a straightforward process that can greatly improve user experience. Whether it’s for displaying notifications, providing quick access to features, or simply adding a professional touch to our app, tray icons are a valuable addition to any desktop application.
With Electron.js, implementing tray icons becomes seamless, allowing us to focus on crafting an exceptional user experience for our desktop application. So why not enhance our Electron.js app with a sleek tray icon for innovative ideas?
Hope you enjoy that.
By Asahi
waithaw at 2024年02月27日 10:00:00